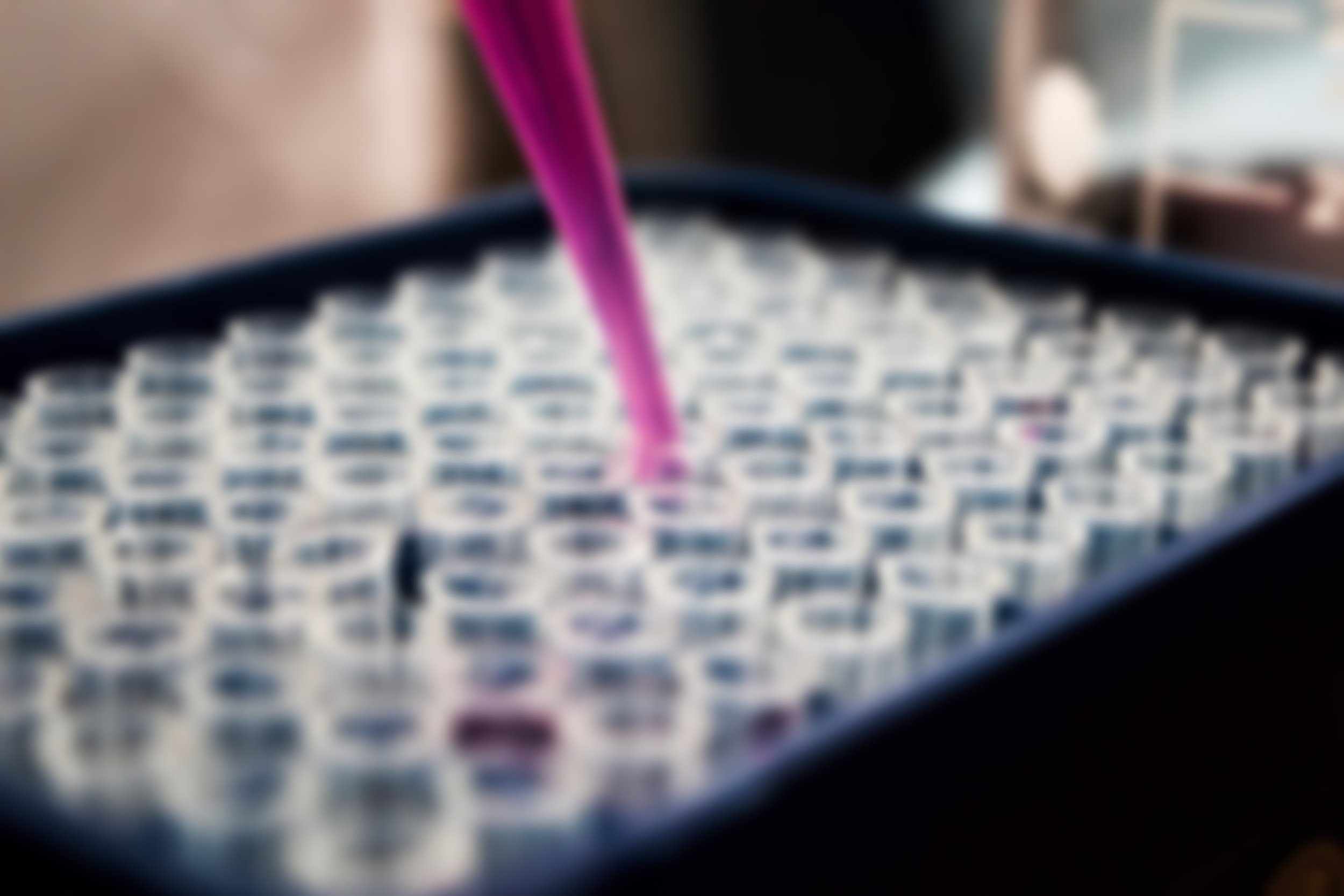
Silverberry Leanring Health System Platform
Silverberry Learning Health System is designed to improve individuals' health and personalized care through the integration of knowledge-generation processes into daily practice.
The methodology employed by Silverberry Health involves the systematic integration of internal data and external evidence, analyzing patient data to look for trends to predict effective treatment plans, and facilitate evidence-based care in real-time.
Silverberry Learning Health System Model
In the United States alone, every year about 200,000 people die from the wrong diagnosis.
Geoffrey Hinton, renowned as the 'Godfather of AI,' shares his insights on the potential of AI in healthcare. The Silverberry Learning Health System embodies this vision, utilizing AI to enhance healthcare outcomes.
Disclaimer: Work on the Silverberry Learning Health System began long before discovering this interview.
What is Silverberry Learning Health System?
Silverberry Learning Health System (SLHS) is an integrated framework that utilizes real-time health data to improve patient care and outcomes. It continuously learns from each patient encounter through advanced AI and data analytics, evolving to provide personalized, evidence-based healthcare. This system represents the convergence of clinical practice, research, and technology to create a dynamic, patient-centric model of healthcare.
How Silverberry Learning Health System Helps Physicians and Patients to Achieve Better Health Outcomes?
For physicians, an LHS offers decision support tools that draw on vast datasets to inform treatment plans, reducing uncertainties and improving care efficiency. Patients benefit from a system tailored to their unique genetic makeup and lifestyle, leading to more effective treatments and proactive health management. Together, these advantages foster a collaborative environment where data-driven insights lead to superior health outcomes and enhanced patient experiences.
Components of the Silverberry Learning Health System
Precision Health Data: Integrating patient information, including genetic profiles, for personalization.
Electronic Health Records (EHR): Integration with the existing digital health and medical records.
Scientific Literature: Current research data informing predictive models and treatment options, annotated and summarized by AI.
AI/ML Training and Prediction Model: algorithms, methods, and techniques to interpret data to information Knowledge Graph to convert data to knowledge.
Knowledge Graph: The core system that learns and adapts to provide personalized health insights. A dynamic, interconnected model that learns continuously and evolves with new data.
Continuous Monitoring: Real-time health tracking to inform and adjust treatment plans, integrated with sensors and wearables to receive aggregated biomarkers.
Patient Engagement with AI Health Companion (Pingoo.AI): Interactive support and health education for patients through AI.
Why should you consider a learning health system?
Silverberry Health can be utilized by various healthcare organizations, including hospitals, clinics, research institutions, and senior living organizations.
It is particularly beneficial for patients with chronic diseases such as diabetes, chronic kidney disease, and cancer and those concerned with longevity and healthy aging.
The platform's API allows integration with electronic health record systems and medical devices, enabling real-time data gathering, analysis, and reporting.
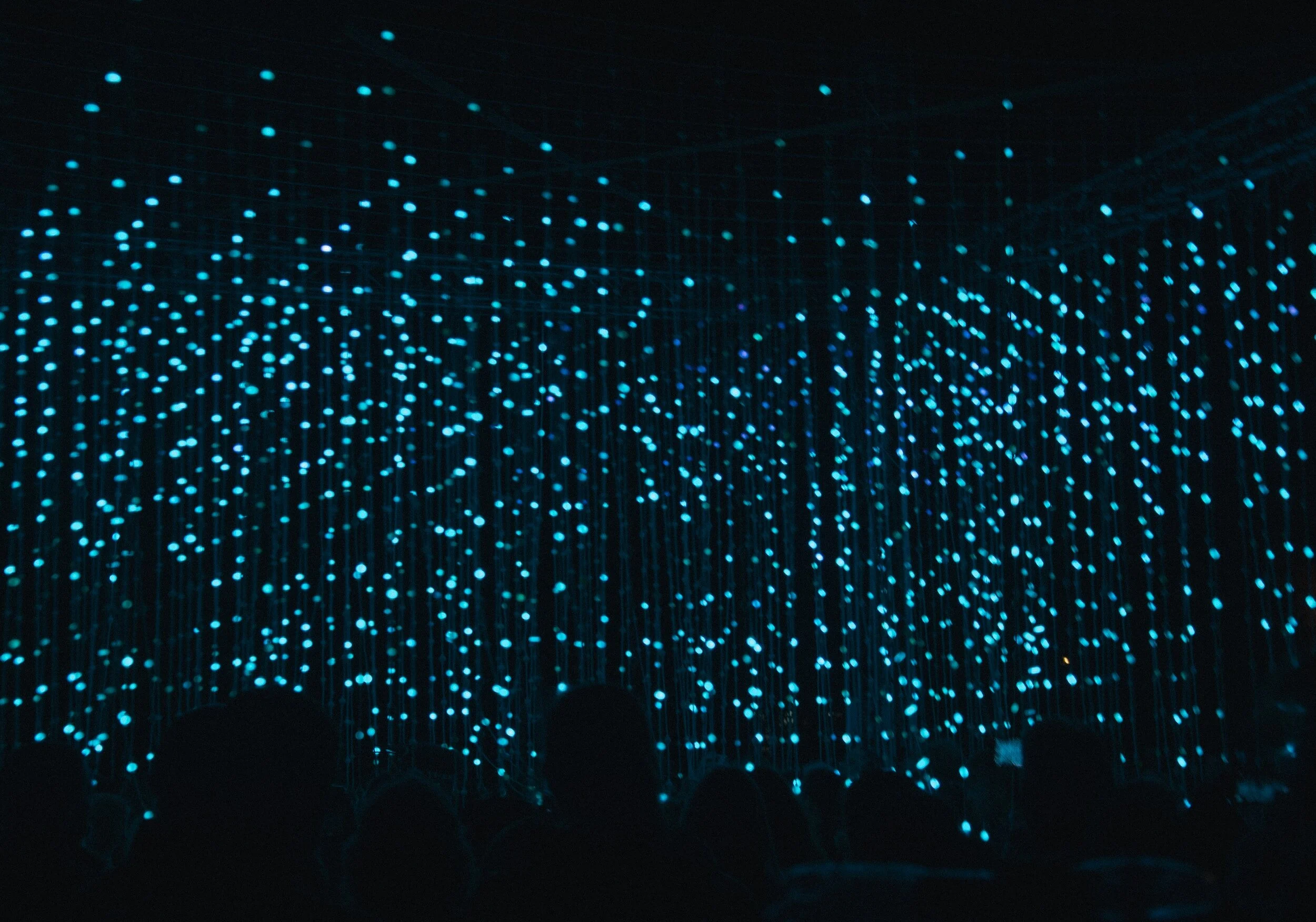
Join the AI movement Today!
Empower your healthcare organization with the future of personalized health. Contact Silverberry today to integrate Silverberry Learning Health System into your practice and join the forefront of precision health.
Profiling, Recommendation, Assessment
Through its user-friendly app, Silverberry delivers personalized health education using micro-learning. It engages users daily, creating a Knowledge Profile and personalized learning path to bridge knowledge gaps and improve health outcomes.